In the world of statistics, hypothesis testing plays a crucial role in determining the validity of a research claim. Two essential components of hypothesis testing are p-value and sample size. The p-value measures the probability of observing the results, assuming the null hypothesis is true, while the sample size determines the reliability of the test. In this article, we will explore the five ways hypothesis testing impacts p-value and sample size.
The Importance of P-Value in Hypothesis Testing

P-value is a critical component of hypothesis testing, as it helps researchers determine the significance of their results. A small p-value (typically less than 0.05) indicates that the observed data would be unlikely under the null hypothesis, leading to the rejection of the null hypothesis. On the other hand, a large p-value suggests that the data are consistent with the null hypothesis, and it should not be rejected.
1. Type I Error and P-Value
Type I error occurs when a true null hypothesis is rejected. The p-value is directly related to the probability of Type I error. A smaller p-value indicates a lower probability of Type I error, while a larger p-value increases the risk of Type I error. Therefore, researchers must carefully choose the significance level (alpha) to minimize the risk of Type I error.
The Role of Sample Size in Hypothesis Testing

Sample size is another crucial aspect of hypothesis testing, as it affects the reliability of the test results. A larger sample size provides more precise estimates of the population parameters, increasing the test's power to detect statistically significant differences.
2. Sample Size and Test Power
The power of a test is its ability to detect statistically significant differences when they exist. A larger sample size increases the test power, as it provides more precise estimates of the population parameters. However, increasing the sample size beyond a certain point may not significantly improve the test power, as the law of diminishing returns applies.
The Relationship Between P-Value and Sample Size

The p-value and sample size are closely related. A larger sample size can result in a smaller p-value, as the test becomes more sensitive to detecting statistically significant differences. However, this relationship is not always linear, and the effect of sample size on p-value depends on the specific research question and study design.
3. The Impact of Sample Size on P-Value
A larger sample size can lead to a smaller p-value, as the test becomes more powerful in detecting statistically significant differences. However, this effect can be mitigated by the research question and study design. For example, if the effect size is small, a larger sample size may be required to detect statistically significant differences.
Common Misconceptions About P-Value and Sample Size

Despite their importance in hypothesis testing, p-value and sample size are often misunderstood. One common misconception is that a smaller p-value always indicates a more significant result. However, this is not necessarily true, as the p-value depends on the sample size and research question.
4. Misinterpreting P-Value and Sample Size
Another common misconception is that a larger sample size always leads to a more significant result. However, this is not always true, as the effect of sample size on p-value depends on the specific research question and study design. Additionally, a larger sample size can lead to the detection of statistically significant differences that are not practically significant.
Best Practices for Hypothesis Testing
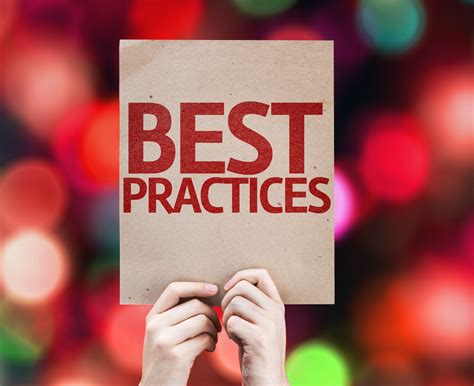
To ensure accurate and reliable results, researchers must follow best practices for hypothesis testing. This includes carefully choosing the significance level, selecting an appropriate sample size, and considering the research question and study design.
5. Avoiding Common Pitfalls
Researchers must avoid common pitfalls in hypothesis testing, such as misinterpreting p-value and sample size. Additionally, they must be aware of the limitations of hypothesis testing and consider alternative methods, such as confidence intervals and Bayesian analysis.
Gallery of Hypothesis Testing and P-Value:






FAQ:
What is the significance of p-value in hypothesis testing?
+The p-value measures the probability of observing the results, assuming the null hypothesis is true. A small p-value indicates that the observed data would be unlikely under the null hypothesis, leading to the rejection of the null hypothesis.
How does sample size affect the p-value?
+A larger sample size can result in a smaller p-value, as the test becomes more sensitive to detecting statistically significant differences.
What are common misconceptions about p-value and sample size?
+Common misconceptions include assuming that a smaller p-value always indicates a more significant result and that a larger sample size always leads to a more significant result.
We hope this article has provided you with a comprehensive understanding of the impact of hypothesis testing on p-value and sample size. By carefully considering these factors, researchers can ensure accurate and reliable results. Share your thoughts on the importance of hypothesis testing in the comments below!